Demystifying Data Science: Unraveling the Power of Data
Demystifying Data Science: Unraveling the Power of Data
Introduction:
In the digital age, data has emerged as a ubiquitous asset, fueling innovations, driving decision-making, and reshaping industries. At the forefront of harnessing the potential of data stands the interdisciplinary field of data science. This blog delves into the essence of data science, elucidating its key concepts, methodologies, and transformative impact on diverse domains.
Understanding Data Science:
Data science is an interdisciplinary field that encompasses various techniques, tools, and methodologies for extracting insights and knowledge from data. At its core, data science integrates elements from computer science, statistics, mathematics, and domain-specific expertise to analyze complex datasets and derive actionable insights. It encompasses a spectrum of activities, including data collection, preprocessing, analysis, modeling, visualization, and interpretation.

Data Collection: Data science begins with the acquisition of relevant data from various sources, including databases, sensors, social media, and IoT devices. The quality and quantity of data play a crucial role in the effectiveness of subsequent analyses and insights.
Data Preprocessing: Raw data often require preprocessing to clean, transform, and format it for analysis. This step involves tasks such as data cleaning, missing value imputation, normalization, and feature engineering to ensure data quality and relevance.
Exploratory Data Analysis (EDA): EDA involves visualizing and summarizing data to gain insights into its underlying patterns, trends, and relationships. Techniques such as descriptive statistics, data visualization, and dimensionality reduction help analysts understand the structure and characteristics of the data.
Statistical Modeling and Machine Learning: Data science leverages statistical techniques and machine learning algorithms to build predictive models, classify data, cluster patterns, and uncover hidden structures. Supervised learning, unsupervised learning, and reinforcement learning are common paradigms employed for solving diverse tasks, including regression, classification, clustering, and recommendation.
Model Evaluation and Interpretation: Evaluating model performance and interpreting results are critical steps in data science to ensure the reliability and validity of insights. Techniques such as cross-validation, confusion matrices, and feature importance analysis help assess model accuracy, generalization, and robustness.
Communication and Visualization: Effective communication of insights is essential for facilitating decision-making and driving organizational impact. Data visualization techniques, such as charts, graphs, and dashboards, enable analysts to convey complex information in a clear, intuitive manner to stakeholders.
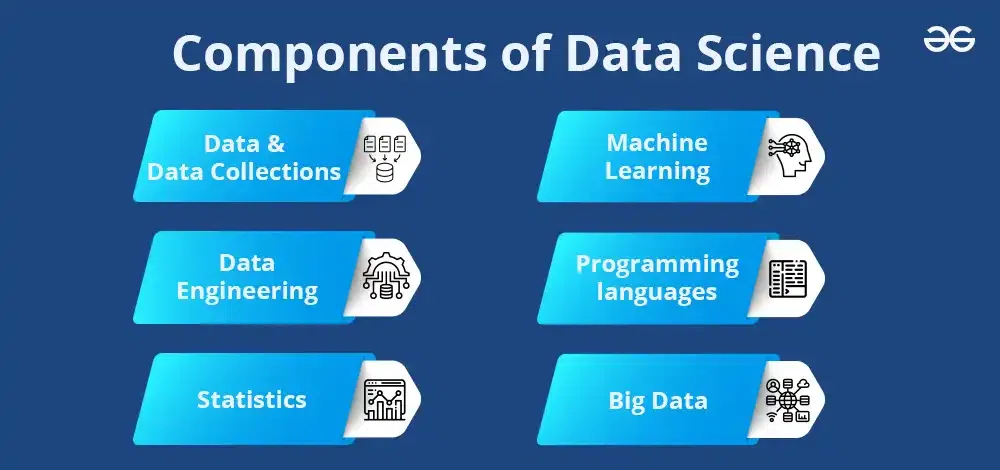
Applications of Data Science: Data science finds applications across a wide range of domains, including finance, healthcare, marketing, retail, transportation, and cybersecurity. In finance, data science is utilized for fraud detection, risk assessment, algorithmic trading, and portfolio optimization. In healthcare, it powers personalized medicine, disease prediction, medical imaging analysis, and drug discovery. Similarly, in marketing, data science drives customer segmentation, sentiment analysis, recommendation systems, and campaign optimization.

Challenges and Future Directions:
Despite its transformative potential, data science faces challenges related to data privacy, security, ethics, and interpretability. Addressing these challenges requires interdisciplinary collaboration, regulatory frameworks, and ethical guidelines to ensure responsible and ethical use of data. Moreover, advancements in areas such as deep learning, natural language processing, and federated learning hold promise for unlocking new frontiers in data science and driving innovations in AI-driven decision-making.

Comments
Post a Comment