Exploring the Depths of Deep Learning: Unveiling the Future of Artificial Intelligence
Exploring the Depths of Deep Learning: Unveiling the Future of Artificial Intelligence
INTRODUCTION
In the realm of artificial intelligence (AI), deep learning stands as a beacon of innovation, revolutionizing how machines perceive, learn, and comprehend complex data. As an interdisciplinary field merging computer science, mathematics, and neuroscience, deep learning has garnered significant attention for its ability to tackle intricate problems that were once deemed insurmountable. This essay embarks on a journey to explore the intricacies of deep learning, its underlying principles, applications across various domains, and the profound impact it continues to have on society.
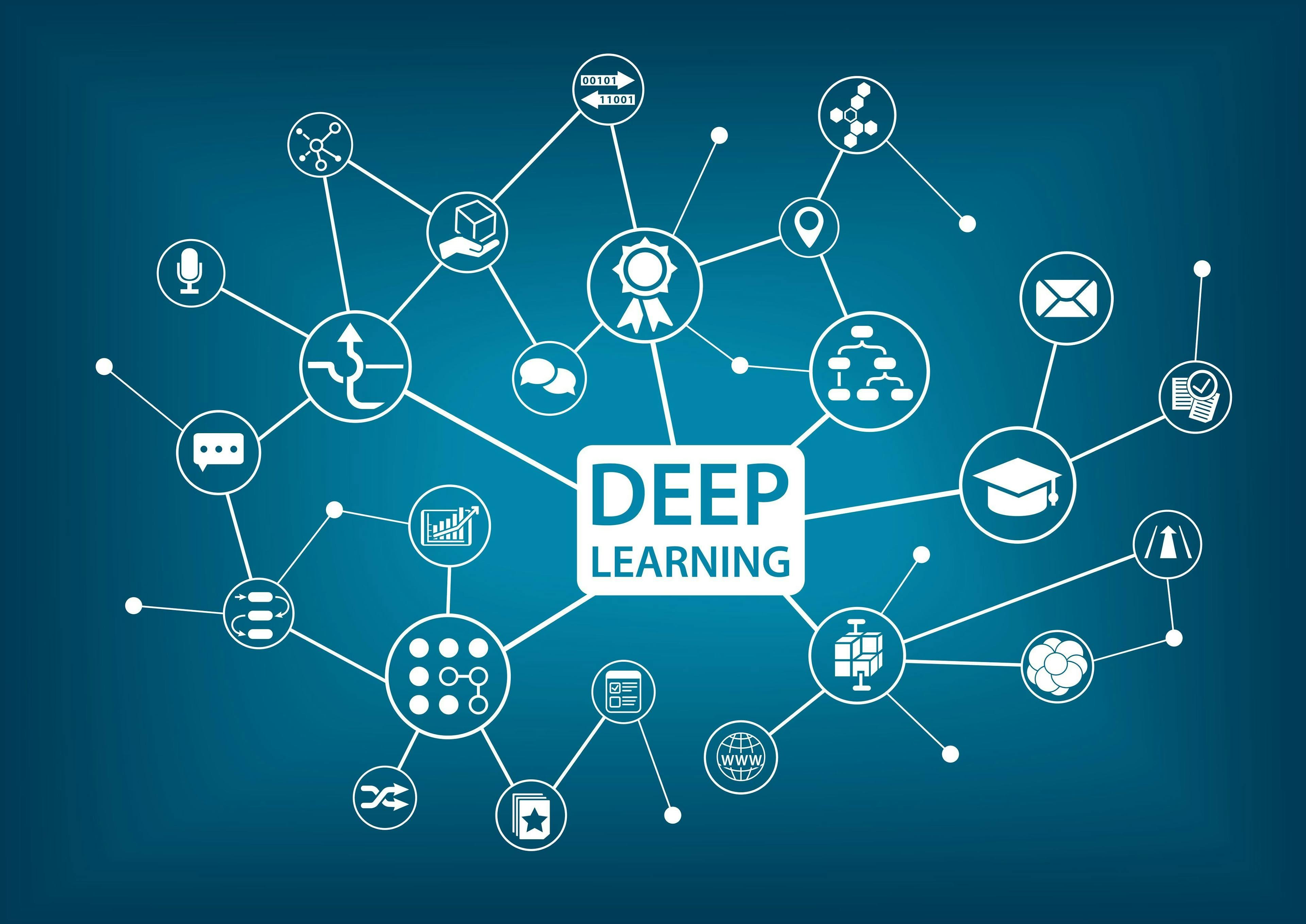
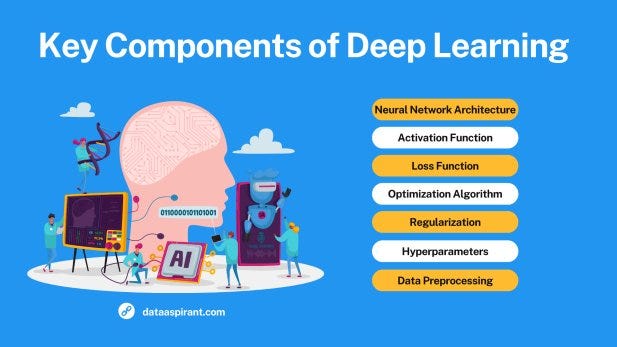
Neural Networks: Deep learning architectures are predominantly built upon artificial neural networks, comprising interconnected layers of neurons. These networks include input, hidden, and output layers, with each neuron performing weighted computations and activation functions to transmit signals across layers.
Convolutional Neural Networks (CNNs): CNNs specialize in processing grid-like data structures, such as images and videos, by employing convolutional layers to extract spatial hierarchies of features. These networks have revolutionized computer vision tasks, including object detection, image classification, and facial recognition.
Recurrent Neural Networks (RNNs): RNNs excel in processing sequential data, such as text, speech, and time-series data, by incorporating feedback loops to capture temporal dependencies. Applications of RNNs span natural language processing, speech recognition, and sentiment analysis.
Applications of Deep Learning:
Healthcare: Deep learning facilitates medical image analysis, disease diagnosis, and drug discovery, revolutionizing healthcare diagnostics and treatment planning. Radiology, pathology, and genomics benefit from deep learning algorithms' ability to identify subtle patterns indicative of diseases.
Autonomous Vehicles: Deep learning powers perception systems in autonomous vehicles, enabling real-time object detection, localization, and path planning. These systems enhance vehicle safety and navigation capabilities, paving the way for widespread adoption of self-driving cars.
Natural Language Processing (NLP): Deep learning techniques, such as recurrent neural networks and transformer models, have propelled advancements in NLP tasks, including machine translation, sentiment analysis, and question answering. Virtual assistants and language translation services exemplify the practical applications of NLP-driven deep learning.
Finance: Deep learning algorithms analyze financial data to forecast market trends, optimize trading strategies, and detect fraudulent activities. These applications empower financial institutions with predictive insights and risk management solutions.
Despite its remarkable achievements, deep learning faces challenges related to data privacy, interpretability, and robustness to adversarial attacks. Addressing these challenges requires interdisciplinary collaborations, ethical considerations, and advancements in model explainability techniques. Furthermore, the future of deep learning entails exploring novel architectures, such as graph neural networks and neuro-symbolic systems, to tackle complex reasoning tasks and bridge the gap between perception and cognition.
Conclusion: In conclusion, deep learning epitomizes the pinnacle of AI innovation, revolutionizing industries
enhancing human capabilities, and reshaping societal paradigms. Its ability to autonomously learn from data, discern intricate patterns, and make informed decisions heralds a future where machines seamlessly interact with and augment human intelligence. As we delve deeper into the realms of deep learning, let us embrace its transformative potential while remaining vigilant of ethical implications and societal repercussions. Deep learning not only empowers us to unlock the mysteries of the digital age but also beckons us to explore the depths of human ingenuity and imagination.
Comments
Post a Comment