Unraveling the Power of Statistics in Data Science
Unraveling the Power of Statistics in Data Science
In the rapidly evolving world of technology and data-driven decision making, data science has emerged as a pivotal field. At its core, data science is about extracting meaningful insights from data to inform decisions, predict trends, and understand patterns. A crucial component of data science is statistics, which provides the theoretical foundation and practical tools necessary for making sense of complex data. This essay explores the power of statistics in data science, highlighting its essential role in data analysis, predictive modeling, and decision making.

.
The Foundation of Data Analysis
Statistics is integral to data analysis, offering methods to summarize, explore, and interpret data. Descriptive statistics, which include measures such as mean, median, standard deviation, and range, provide a way to understand the central tendency and variability within a dataset. These measures help data scientists to quickly grasp the overall distribution and spread of the data, laying the groundwork for more advanced analyses.
Inferential statistics extend the capabilities of data analysis by allowing data scientists to make predictions and inferences about a population based on a sample. Techniques such as hypothesis testing, confidence intervals, and regression analysis enable the determination of relationships between variables and the generalization of findings from a sample to a broader population. This inferential power is essential for making informed decisions in business, healthcare, social sciences, and many other fields.
.png)
Enabling Predictive Modeling
Predictive modeling is a cornerstone of data science, and statistics provides the mathematical underpinnings for these models. Regression analysis, a fundamental statistical technique, helps in understanding the relationship between dependent and independent variables. Linear regression models, for instance, are used to predict continuous outcomes, while logistic regression is used for binary classification problems.
Beyond traditional regression methods, advanced statistical techniques like time series analysis and survival analysis are critical for specific types of data. Time series analysis is used to analyze temporal data, identifying trends, seasonal patterns, and cyclic behaviors, which is invaluable in fields like finance and economics. Survival analysis, on the other hand, focuses on time-to-event data and is extensively used in clinical trials and reliability engineering.
Machine learning, a subfield of data science, heavily relies on statistical principles. Algorithms such as decision trees, random forests, and support vector machines are grounded in statistical theories. Even neural networks, which power deep learning models, incorporate statistical concepts to optimize their performance through techniques like gradient descent.
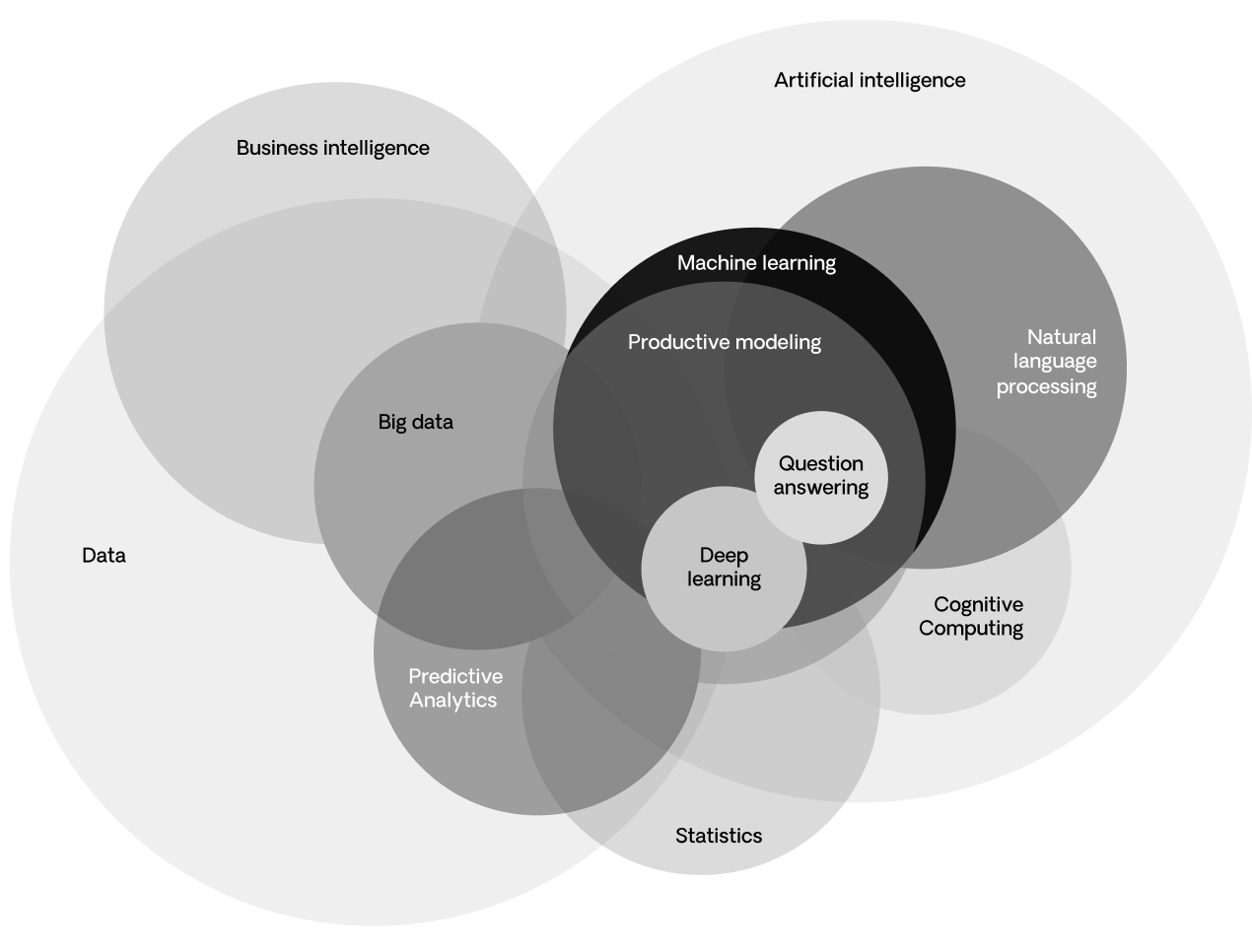
Guiding Decision Making
Statistics play a crucial role in guiding decision-making processes by providing a framework for assessing risks, benefits, and uncertainties. In business, statistical methods are used to conduct market analysis, forecast sales, and optimize operational processes. A/B testing, a statistical method, is widely used in marketing and product development to compare the effects of different interventions and determine the most effective strategy.
In healthcare, statistics inform clinical decisions and public health policies. The design and analysis of clinical trials rely on statistical methods to evaluate the efficacy and safety of new treatments. Epidemiological studies use statistics to track disease outbreaks, identify risk factors, and develop strategies for prevention and control.
Furthermore, statistics help in evaluating the performance of predictive models and ensuring their reliability. Metrics such as accuracy, precision, recall, and F1 score are used to assess the effectiveness of classification models. For regression models, metrics like R-squared, mean absolute error, and root mean square error are crucial for evaluating model performance and making necessary adjustments.
Challenges and Ethical Considerations
While statistics are powerful, their application in data science is not without challenges. Issues such as data quality, sample bias, and overfitting can significantly impact the reliability of statistical analyses and models. Ensuring that data is representative and models are validated rigorously is essential for producing trustworthy results.
Ethical considerations also play a vital role in the application of statistics in data science. The misuse of statistical methods can lead to misleading conclusions and potentially harmful decisions. It is imperative for data scientists to adhere to ethical standards, ensuring transparency, accountability, and fairness in their analyses.
Conclusion
In conclusion, the power of statistics in data science cannot be overstated. From providing the foundational tools for data analysis to enabling sophisticated predictive models and guiding critical decision-making processes, statistics are indispensable. As data continues to grow in volume and complexity, the role of statistics in unraveling insights and driving innovation will only become more significant. By addressing challenges and upholding ethical standards, data scientists can harness the full potential of statistics to make informed, impactful decisions across various domains.
Compiled by: Pratiksha Bisht
Comments
Post a Comment